
One of the primary advantages of integrating KGs with RAG is the improvement in contextual relevance. KGs provide a structured framework that ensures retrieved information is not only relevant but deeply contextual. This is particularly crucial when dealing with complex queries that require a nuanced understanding of relationships between entities. By leveraging the interconnected nature of data within KGs, RAG systems can efficiently handle intricate questions, offering responses that are more aligned with the user's intent.
The combination of KGs and RAG also addresses one of the most pressing challenges faced by LLMs: hallucination. LLMs, when left to their pre-trained parameters alone, often generate plausible-sounding but factually incorrect responses. By grounding LLM outputs in the factual information stored in KGs, the risk of hallucination is significantly reduced. This is especially valuable in domains where accuracy is paramount, such as healthcare or technical support.
Understanding GraphRAG
Contrary to the traditional semantic-search approaches using plain text snippets, GraphRAG is a structured, hierarchical approach to the Retrieval Augmented Generation (RAG). The GraphRAG process involves extracting a knowledge graph from the raw text, building a community hierarchy, generating summaries for these communities, and then leveraging these structures when performing RAG-based tasks. It also allows LLMs to access external data sources without requiring retraining. While RAG typically relies on vector databases for storing and retrieving information, GraphRAG takes this further by incorporating graph databases into the mix.
The key components of GraphRAG include:
- Knowledge Graphs are strong tools that capture intricate relationships between different entities, providing a valuable and comprehensive context for AI models to use in their decision-making processes. They can represent information in a way that helps AI systems to understand and interpret complex relationships.
- Vector Databases are designed for efficient storage and retrieval of unstructured data, utilizing mathematical representations to process and manage data in a way that optimizes storage and retrieval processes.
- Graph Databases specifically store interconnected and structured information. They are ideal for representing relationships between different data points and provide a robust method for managing complex interconnections.
- Retrieval Mechanisms encompass advanced algorithms that combine vector and graph search techniques to find the most pertinent information. These mechanisms are essential for leveraging the advantages of both data storage methods and ensuring that AI systems can effectively retrieve valuable information when needed.
By combining these elements, GraphRAG enables LLMs to access a more comprehensive and contextually rich dataset, leading to more accurate and nuanced responses.
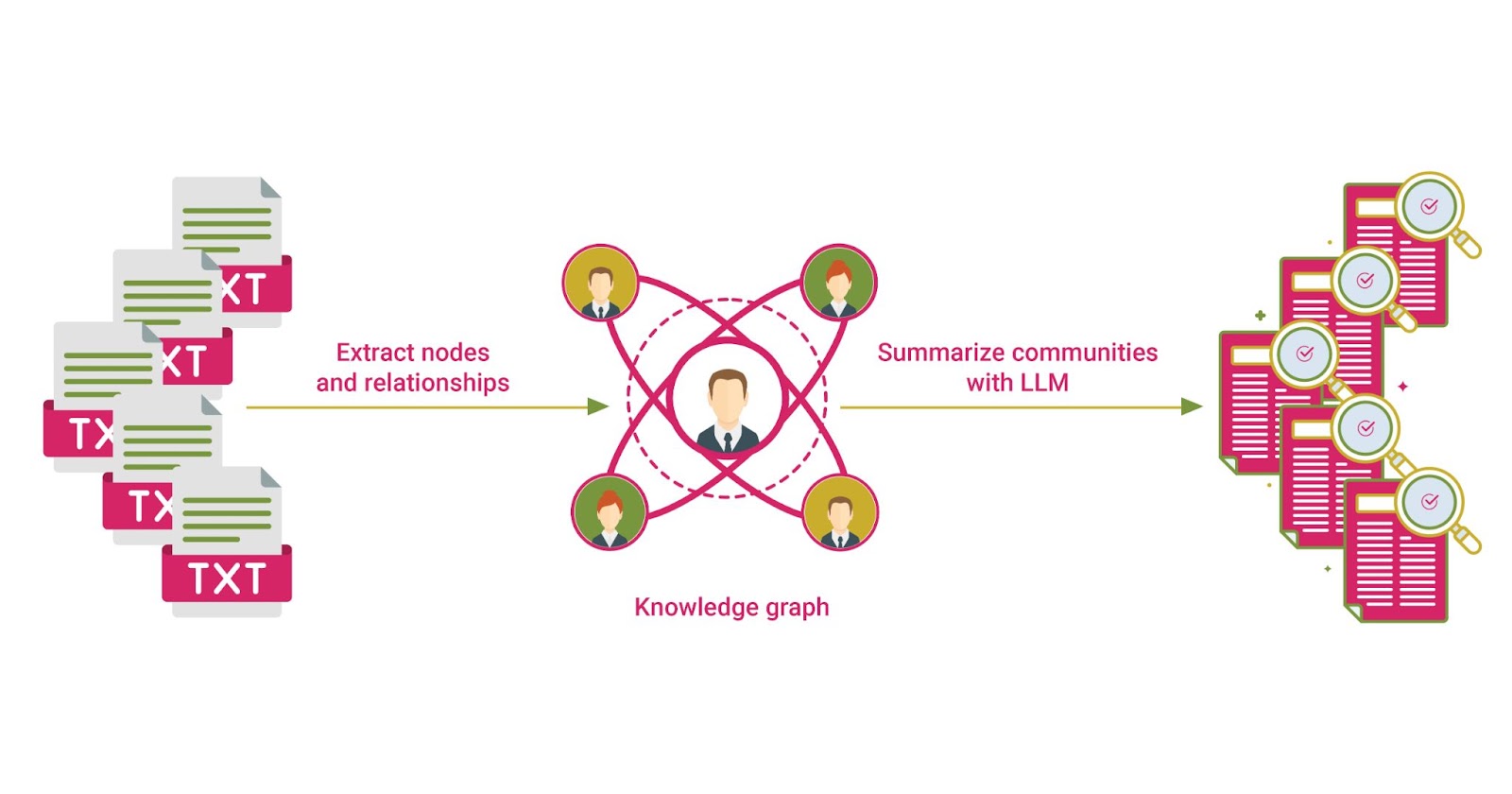
Advantages of GraphRAG over Vector RAG
This strategy is a prime component of most LLM-based tools, and most RAG approaches (which we refer to as Baseline RAG) use vector similarity as the search technique. As for private datasets—material that an LLM is not trained on and has never seen before—like an organization's confidential research, business records, or emails, RAG approaches have demonstrated promise in supporting LLMs in making sense of the data.
GraphRAG offers several significant advantages over traditional Vector RAG approaches:
- Improved Accuracy: When reasoning about complex material, GraphRAG significantly improves question-and-answer performance with knowledge graphs
- Enhanced Explainability: Knowledge graphs facilitate tracing the reasoning behind AI-generated responses, improving transparency and trust.
- Real-time Data Integration: GraphRAG enables seamless incorporation of up-to-date information, ensuring that AI responses remain current and relevant.
- Reduced Hallucinations: Anchoring responses in factual, structured data significantly decreases the likelihood of AI hallucinations or fabricated information.
- Complex Reasoning Capabilities: GraphRAG empowers LLMs to perform more intricate reasoning tasks by leveraging the relationships captured in knowledge graphs.
Environmental Benefits of GraphRAG
GraphRAG offers climate benefits by reducing the environmental impact of GenAI applications. While that is often overlooked, it is achieved through two main factors:
- Improved Retrieval Accuracy: GraphRAG more accurately identifies relevant information, leading to reduced unnecessary data processing. This results in fewer computations and lower energy consumption.
- Reduced Context Size: Knowledge graphs' structured nature allows for efficient representation of information. As a result, LLMs can work with smaller, more focused contexts, thus reducing computational requirements.
These efficiency improvements directly contribute to a lower carbon footprint for GenAI applications. Considering concerns about the environmental impact of the AI industry, technologies like GraphRAG offer a path towards more sustainable AI practices.
The Future of GraphRAG
In conclusion, the integration of knowledge graphs with RAG represents a significant advancement in AI technology. By combining the linguistic prowess of LLMs with the structured knowledge representation of KGs, we can create AI systems that are not only more accurate and contextually aware but also more transparent and trustworthy. As this field continues to evolve, we can expect to see even more innovative applications that leverage the synergy between knowledge graphs and RAG, pushing the boundaries of what's possible in natural language processing and AI-driven decision-making.